Team I Comparative Genomics Group
Members: Frank Ambrosio, Vasanta Chivukula, Seonggeon Cho, Siarhei Hladyshau, Junyu Li, Yiqiuyi Liu, Yihao Ou, Hunter Seabolt and Qinyu Yue.
Background
Klebsiella is a gram negative, non-motile rod shaped bacteria found singly, in pairs or in chains. The bacterium is encapsulated which helps evade phagocytosis. Klebsiella are facultative anaerobes and can perform both anaerobic respiration and fermentation. Species of Klebsiella, especially Klebsiella pneumoniae, are known to cause respiratory tract infections such as pneumonia and urinary tract infections. Antibiotic resistance has been noted in this genus for decades but heteroresistance phenomenon (first documented in 1946) has been recently observed in this bacteria. Genetically identical, but phenotypically distinct, subpopulation of colistin-resistant Klebsiella spp. are of interest in the current study. Comparing the genomes of the isolates that are heteroresistant to other genomes helps us identify specific genes or parts of genes that attribute this feature to the isolate.
Comparative genomics as the name suggests is comparing the similarities and differences between two or more genomes of the same or different species. This provides with a detailed view of how organisms are related to each other at the genetic level. It is a powerful tool in understanding evolution. Advances in the field of comparative genomics will have implications in fields as disparate as ecology, agriculture to biotechnology and human health. This tool can help us identify the similarities/differences between the heteroresistant and other subpopulations.
Objective
The goal of this project is to understand the source of Colistin heteroresistance in Klebsiella spp. To achieve this goal, the class was tasked with assembling reads obtained from David Weiss’ lab, predict and annotate the genes, compare the genomes and create a predictive webserver that would serve as an online resource for these samples.
As a part of the Comparative Genomics group, our objective is to compare the annotated genomes provided by the annotation group with other genomes to identify the genetic determinants that could be a potential cause for colistin heteroresistance in the samples.
Colistin Resistance
Colistin is an antibiotic produced by Bacillus polymyxa spp colistinus, discovered in 1940. Due to its high toxicity (nephrotoxic), it was replaced by newer antibiotics during the 1980s. Due to growing resistance of bacteria to various antibiotics, there is a renewed interest in colistin, especially to treat multi-drug resistance gram negative bacteria.
Colistin is a cationic antibody composed of heptapeptide covalently attached to a fatty acyl chain.
Colistin: Mode of action
-
Bacterial LPS
-
Colistin displaces the divalent cations
-
This destabilizes the outer membrane
Colistin Resistance
There are various modes of bacterial resistance to polymyxin antibiotics (the group that colistin belongs). It can be via porin proteins (reported in Salmonella), efflux pumps (reported in Yersinia and Klebsiella), capsule (Klebsiella), LPS modification. In case of Klebsiella there is an interplay of various modes of resistance including LPS modification. Additionally, heteroresistance, a variable response showed by a subpopulation to a specific antibiotic, is observed in Klebsiella. Many mechanisms have been attributed to heteroresistance including a mutation in the gene of the PhoP protein involved in the PhoP/PhoQ pathway to gain resistance to colistin. According to David Weiss, some of the samples provided for this project have been found to be genetically identical, heteroresistant subpopulations, lacking the above mentioned mutation in the PhoP protein.
Methods
MASH Principle Component Analysis plots
To study similarity of samples we calculated MASH distances between all assembled genomes. After that we performed Principal Component Analysis to understand variation of samples in population. First two principal components showed clear separation of different species (K. oxytoca and K. variicola), however, no clustering was observed for phenotype (heteroresistant, resistant and succeptable). In addition, K. variicola genomes showed separation in two subgroups, again proving that our population is stratified and additional correction methods are needed to represent valid variant calling.
To analyze variability across samples we selected a group of four closely related genomes from the dendrogram, that have different phenotypes. Note, that similarity of these samples was also proved by tSNE plot of MASH distances (see MASH tSNE). We used MAUVE to obtain whole genome alignment of these samples. Further analyses showed regions of relatively high variability, proving that phenotype may depend both on single SNPs as well as on larger variants like indels or presence or absence of genes. This provides reasoning for selecting tools for variant identification as depicted by our pipeline.
MASH hierarchical cluster
MASH tSNE
Multiple Alignment of the 4 genomes
Pipeline for the project
Results
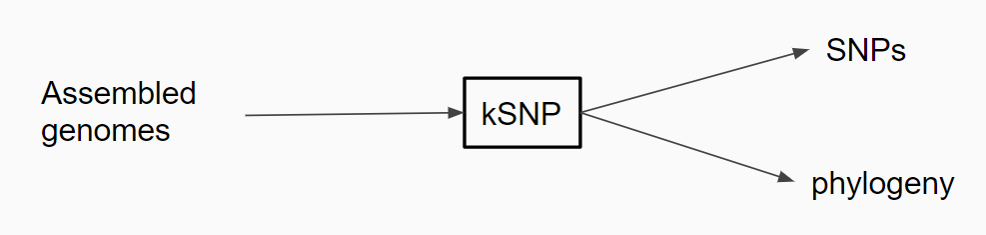
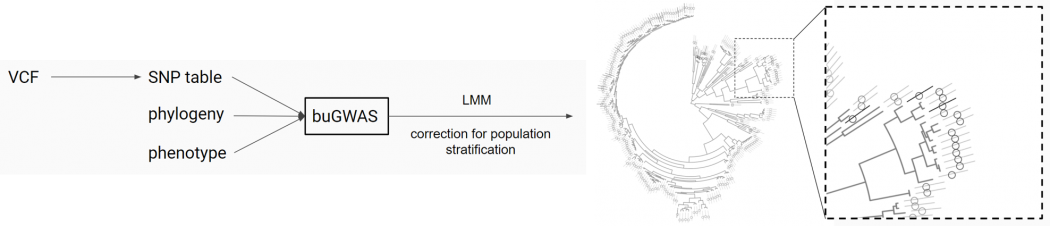
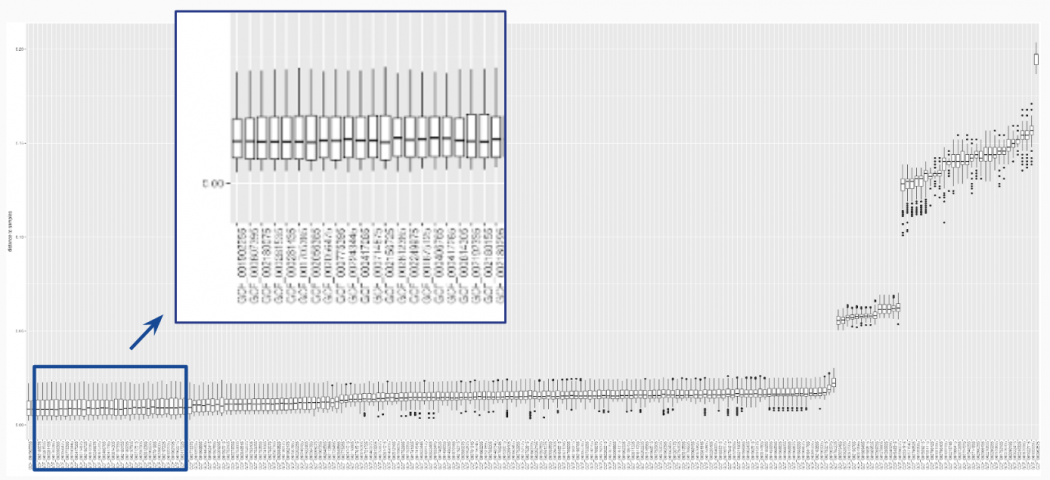
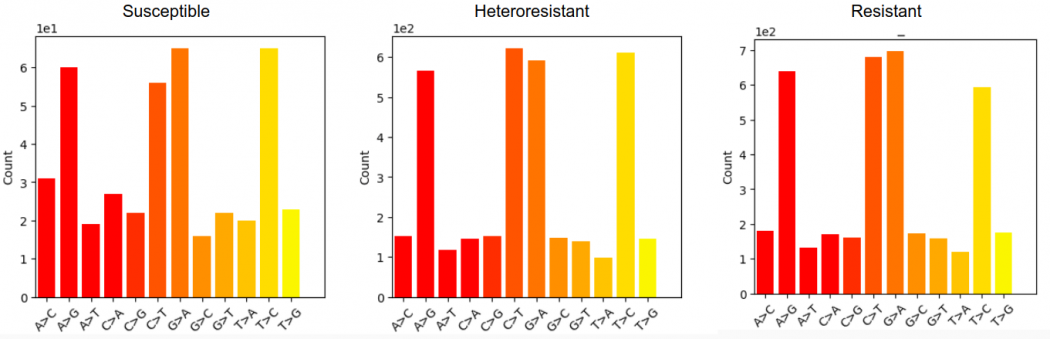

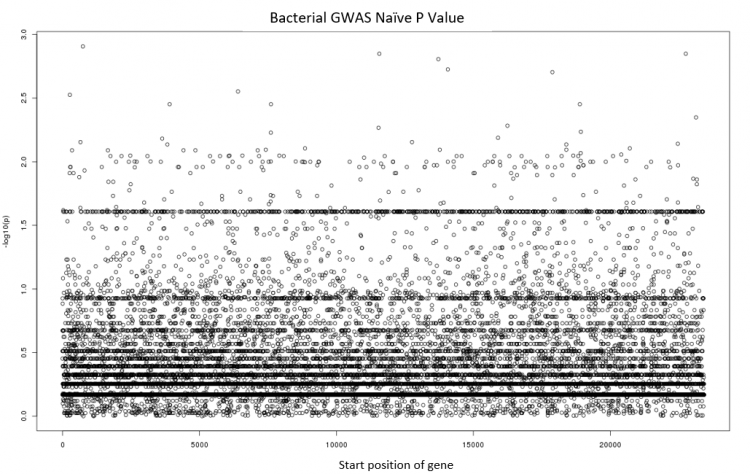
- Manhattan Plot with naïve P value. X axis is the start position of gene, Y axis is the -log10(p). Case: Hetero-resistant (21) Control: Susceptible and Resistant (223)
In order to correct the P value with respect to the multi comparisons, we chose to adopt the Benjamini–Hochberg procedure, to control the false discovery rate. Benjamini–Hochberg procedure first puts the individual P values in order, from smallest to largest. The smallest P value has a rank of i=1, then next smallest has i=2, etc. Compare each individual P value to its Benjamini-Hochberg critical value, (i/m)Q, where i is the rank, m is the total number of tests, and Q is the false discovery rate you choose. The largest P value that has P<(i/m)Q is significant, and all of the P values smaller than it are also significant, even the ones that aren't less than their Benjamini-Hochberg are critical values.
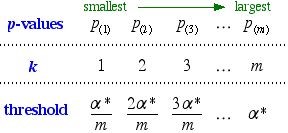
After the correction is applied, no significant results have been returned. There is not enough evidence to find any significant correlation between a certain gene with certain trait.
Ongoing Research
SNP analysis of the Klebsiella spp. bacterial genomes yielded no unique identifier for each of our phenotypic classes. This indicates that our heteroresistant phenotype and our resistant phenotype contain the same sequences.
Plasmids contain a variety of AMR genes. The MCR family of genes all confer resistance to colistin. Annotations for MCR genes were made on genomes in all three phenotype groups.
The AMR genes on plasmids of Klebsiella have particularly high stability which can be shown by the fact that even years after extended-spectrum cephalosporins stopped being used to treat Extended spectrum β-lactamase producing Klebsiella spp., it is still possible to find the genes which confer resistance to this drug on their plasmids.
These facts lead one to believe that the resistant subpopulation may be due to copy number variation of AMR genes.
First, the average kmer depths for colistin resistance conferring genes from the CARD database were determined using the program STing in GDETECT mode. The results were visualized for each isolate. The patterns in the plots were consistent across all three phenotype groups. Upon seeing the same patterns in these graphs across phenotype classes it was determined that per nucleotide coverage depth statistics would provide much greater resolution. It may also be possible that copy numbers of other genes on the plasmid may be effecting expression.
To investigate this further the plasmids found in the set of isolates were assembled using plasmidSpades and the gene sequences were extracted to form the pan-plasmidome. Using the pan-plasmidome as a reference, the per base nucleotide depths will be determined. We hope to find a clear distinction between phenotype groups based on copy number variation both of the whole plasmid, and copy number variation of genes on individual plasmids. File:Wiki comp gen frank.pdf
References
- Andrew J. Page, Carla A. Cummins, Martin Hunt, Vanessa K. Wong, Sandra Reuter, Matthew T. G. Holden, Maria Fookes, Daniel Falush, Jacqueline A. Keane, Julian Parkhill, Roary: Rapid large-scale prokaryote pan genome analysis, Bioinformatics, (2015). doi: http://dx.doi.org/10.1093/bioinformatics/btv421
- Brynildsrud O, Bohlin J, Scheffer L, Eldholm V. Rapid scoring of genes in microbial pan-genome-wide association studies with Scoary. Genome Biol. 2016;17:238 DOI: 10.1186/s13059-016-1108-8
- Fadista, J., Manning, A. K., Florez, J. C., & Groop, L. (2016). The (in)famous GWAS P-value threshold revisited and updated for low-frequency variants. European Journal of Human Genetics, 24(8), 1202-1205. doi:10.1038/ejhg.2015.269
- Falush, D. (2016) Bacterial genomics: Microbial GWAS coming of age. Nature Microbiology 1, 16059
- Gardner, S. N., Slezak, T., & Hall, B. G. (2015). kSNP3. 0: SNP detection and phylogenetic analysis of genomes without genome alignment or reference genome. Bioinformatics, 31(17), 2877-2878
- https://github.com/sgearle/bugwas/tree/master/bugwas
- Ondov, B. D., Treangen, T. J., Melsted, P., Mallonee, A. B., Bergman, N. H., Koren, S., and Phillippy, A. M. (2016) Mash: fast genome and metagenome distance estimation using MinHash. Genome Biology 17, 132
- Olson, Nathan D., et al. "Best practices for evaluating single nucleotide variant calling methods for microbial genomics." Frontiers in genetics 6 (2015): 235.
- Power RA, Parkhill J, de Oliveira T., Microbial genome-wide association studies: lessons from human GWAS. Nat Rev Genet. 2017 Jan;18(1):41-50. doi: 10.1038/nrg.2016.132. Epub 2016 Nov 14.