Team II Comparative Genomics Group
Introduction
Background
Comparative genomics is the study of comparing genome sequences to better understand the structure and function of genes. This field has explored areas ranging from organism development and behavior to metabolism and susceptibility to disease.
Fosfomycin
Fosfomycin is a natural antibacterial produced by various Streptomyces and Pseudomonas species. It is the only antibiotic currently in clinical use that targets a Mur enzyme. It is broad-spectrum bactericidal antibiotic that can be employed against both Gram-positive and Gram-negative bacteria (i.e. Klebsiella). It interferes with cell wall synthesis, particularly inhibits the initial step involving phosphoenolpyruvate synthetase, as shown below.

Resistance of Fosfomycin involves a wide range of resistance mechanisms. Some of them include reduced uptake, target site modification, expression of antibiotic-degrading enzymes and rescue of the UDP-MurNAc biogenesis pathway (ex. mutation within MurA enzyme).
Objectives
To identify genetic determinants that could be a potential cause for Fosfomycin heteroresistance in the isolates provided.
Data
The following is the metadata of our study:

Whole Genome Approach
The Whole Genome approach to comparative genomics attempts to broadly identify similarities and differences across samples.
Similarity analysis
Identifying similarities among our samples would tell us if phenotypic similarities correlate with overall genome similarity and help us choose representatives.
We computed min-hash distances between all samples with known Antibiotic resistance phenotype and clustered them using complete linkage hierarchical clustering.

The dendrogram indicates that there is no clear demarcation between the different resistance phenotypes, confirming that there is no broad genomic signature that distinguishes the heteroresistant samples from the non-heteroresistant samples.
After removing the outliers, we found a single highly similar cluster of isolates that spanned all 3 phenotypes (Resistant, heteroresistant, susceptible)

These 11 samples have relative mash distances =~ 0.0001, suggesting that any genetic element causing the different antibiotic resistance phenotypes would be caused by the extremely small percentage of differences between these samples. We believe this cluster is a good representative of our complete dataset.
Difference analysis
In order to identify the genomic differences between our samples, we decided to perform a Genome Wide Association Study or GWAS. A GWA study correlates the presence or absence of variants in a genome with the presence or absence of a trait, which in our case is heteroresistance.
We executed a pan-genome GWAS using the tool 'bacterial GWAS'. Bacterial GWAS runs prodigal on assembled contigs to annotate ORFs, and performs CD-HIT clustering to construct a pan-genome. Then it performs a GWAS using a logistic regression model on the given phenotypes - presence or absence of heteroresistance and outputs a list of significant predicted genes along with the frequency of their occurrence in each phenotype. We then BLASTed the predicted genes to identify their function.

Piggy
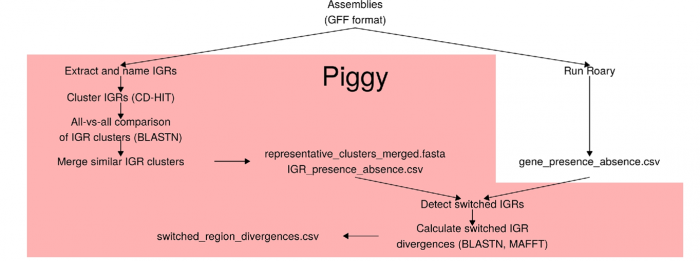
Variation in intergenic regions in bacteria may confer to phenotypes.


Phylogeny Approach
Phylogeny based approaches aim to pair down analysis by focusing on small changes to a directed set of genes between samples. Our group chose to focus on comparison of highly conserved genes and single nucleotide polymorphisms. These approaches attempted to both sequence type and understand the underlying mechanisms of action for heteroresistance in Klebsiella pneumoniae.
Multilocus Sequence Typing (MLST)
Traditional MLST schemes focus on allelic diversity across a small subset of highly conserved genes commonly referred to as housekeeping genes. Compiling allelic variants into compound identification profiles creates unique types which have been shown to have specificity down to the strain level. However, due to housekeeping genes being highly conserved, MLST schemes have difficulty distinguishing between organisms and samples from the same culture.
MLST schemes take years and large amounts of funding to establish and verify. Luckily, an MLST scheme existed previously for Klebsiella pneumoniae. We chose to use this existing scheme along with known phenotypic profiles of our samples in hopes of being able to easily sequence type heteroresistance. STing, an MLST Tool developed by the Jordan Lab at Georgia Tech, was used to quickly assign allelic profiles to our sample set.
The MLST scheme for Klebsiella pneumoniae contains 7 genes and is as follows:
- gapA - Glyceraldehyde-3-phosphate dehydrogenase A
- infB - Translation initiation factor IF-2
- Mdh - Malate dehydrogenase
- Pgi - Glucose-6-phosphate isomerase
- phoE - Outer membrane pore protein E
- rpoB - RNA polymerase subunit B
- tonB - Protein TonB



Single Nucleotide Polymorphism (SNP) Analysis
SNP analysis compares genetic sequences across samples in search of single nucleotide differences. Even single nucleotide changes have been shown to drastically affect genetic expression, transcriptional mechanisms, and protein composition and configuration. Our team chose to compare these sites using kSNP3. kSNP relies on pre-existing string manipulation programs which k-merize the sequences based off of metrics of sequence similarity. kSNP3 also possess the ability to annotate these SNPs found in the genome based off either NCBI reference genomes or provided GenBank files.
Using self-contained scripts, we used a k-mer size of 23 and GenBank files from the Functional Annotation team, we analyzed both the whole sample population and our previously identified cluster of interest. Initial analysis of the trees generated by kSNP3 did not return clear phylogenetic groupings along phenotypic populations. The tree for our cluster of interest is listed below.

After obtaining the trees and SNPs contained within, our focus shifted toward finding SNPs which were homogenous within and unique to our phenotypic populations.

In the end, we were unable to discover any SNPs which were homogenous across our heteroresistant sample population and were not found in susceptible and/or resistant populations. This led us to the conclusion that heteroresistance was not being caused by single nucleotide polymorphisms.
Conclusion
We were unable to identify any genetic determinants of heteroresistace to fosfomycin from our analyses. As the samples are highly genetically similar, any differences we found using GWAS and kSNP analysis did not correlate with resistance phenotype.
References
Castañeda-García A, Blázquez J, Rodríguez-Rojas A. Molecular Mechanisms and Clinical Impact of Acquired and Intrinsic Fosfomycin Resistance. Antibiotics. 2013;2(2):217–236.
Nikolaidis I, Favini-Stabile S, Dessen A. Resistance to antibiotics targeted to the bacterial cell wall. Protein Science. 2014;23(3):243–259.
Kidd TJ, Mills G, Sá‐Pessoa J, Dumigan A, Frank CG, Insua JL, Ingram R, Hobley L, Bengoechea JA. A Klebsiella pneumoniae antibiotic resistance mechanism that subdues host defences and promotes virulence. EMBO Molecular Medicine. 2017;9(4):430–447.
Guo Q, Tomich AD, Mcelheny CL, Cooper VS, Stoesser N, Wang M, Sluis-Cremer N, Doi Y. Glutathione-S-transferase FosA6 of Klebsiella pneumoniae origin conferring fosfomycin resistance in ESBL-producing Escherichia coli. Journal of Antimicrobial Chemotherapy. 2016;71(9):2460–2465.
Thorpe HA, Bayliss SC, Sheppard SK, Feil EJ. Piggy: A Rapid, Large-Scale Pan-Genome Analysis Tool for Intergenic Regions in Bacteria. 2017.
Gardner SN, Slezak T, Hall BG. kSNP3.0: SNP detection and phylogenetic analysis of genomes without genome alignment or reference genome: Table 1. Bioinformatics. 2015;31(17):2877–2878.
Kim M, Oh H-S, Park S-C, Chun J. Towards a taxonomic coherence between average nucleotide identity and 16S rRNA gene sequence similarity for species demarcation of prokaryotes. International Journal Of Systematic And Evolutionary Microbiology. 2014;64(Pt 5):1825–1825.